In ISF Part 1, we discussed the first principles of autonomous vehicle insurance that laid the foundation for the Insurability Sufficiency Framework. This article wants to shed some light on the different parts of the ISF methodology that constitute the ISF Score, which enables us to underwrite autonomous vehicle fleets in a meaningful, reliable, and repeatable way.
We built the ISF Score to be a cornerstone of new insurance programs that will price in the benefits of AVs through autonomous vehicle behavior, safety engineering, and operational metrics. It is no small feat, especially considering the complexity of a given AV system, data sharing implications, and the lack of historical claims data that insurance companies traditionally rely on in their ratings. Thus, the score and the process of generating that score have to be grounded in a sound theory of AV performance to encompass the entirety of the risk assessment but at the same time be practical for both insureds and insurers. All of that went into creating the ISF Score, a crown jewel of the underwriting toolset that we launched with the Singularity Platform this year.
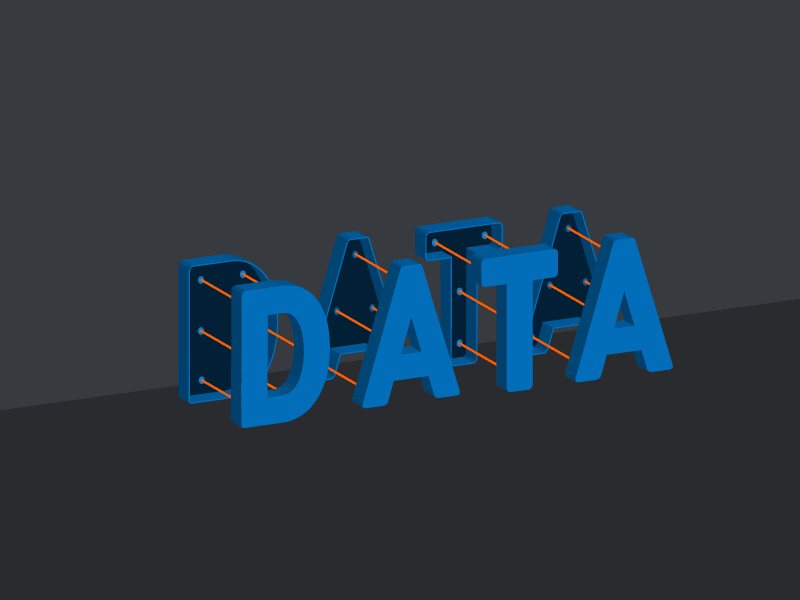
Data
Accessing AV fleet data is of primary importance for risk modeling purposes. Without it, it does not matter how sophisticated a model one can build — at the end of the day, the model has to be tested on the high-quality, real-world data collected from actual AV fleets, and it has to work. Essentially, it is Software 2.0 — “…it turns out that a large portion of real-world problems have the property that it is significantly easier to collect the data (or more generally, identify a desirable behavior) than to explicitly write the program” — but in the risk modeling context applied to autonomy.
In ISF, we focus on four categories of data: exposure, performance, loss, and operating domain. Those are broad categories that contain different metrics and areas of focus. For example, performance data includes both AV behavior metrics as well as operational fleet metrics. The loss category includes not only claim events but also other types of events that could be used for risk modeling. Those categories of data provide us with enough context and granularity to perform a comprehensive risk scoring.
While the data model we use for data sharing has a standardized set of fields regardless of the use case, we realize that there must be room for customization. For example, a robotaxi fleet in a traffic-dense urban environment would likely require a slightly different set of metrics vs. a long-haul, hub-to-hub autonomous trucking deployment. AV developers might also have unique metrics that should be considered in the risk assessment process and be rewarded for providing those.
Most of the data collection happens through Koop API, a secure and cost-effective way to share data from AV fleets. Additionally, we have partnerships with 3rd-party providers of data that complement our direct data sources. Since numerous hazards could cause an accident, we must have the most source-complete model possible, and a way to keep it updated.
As you might notice, our approach to data sharing is 1) flexible enough to evolve with our insureds as they scale and iterate on their own stacks and data collection practices, and 2) thoughtful in a way that it considers all possible friction for AV fleets when it comes to sharing their data externally.

Scoring
With high-quality data, we are able to perform risk scoring. ISF’s analytical engine has two components to it: quantitative and qualitative. The quantitative component is a set of statistical methods that we deploy to spread and assess the performance metrics. It allows us to generate distributions that could be used to gain confidence in a certain level of risk for a given insured or for a given deployment. The qualitative component dives into the custom risk surveys that we developed for our customers and the safety engineering of each AV company. Since ISF is safety standard agnostic, we can interpret and incorporate all kinds of safety assessments into ISF. Safety is rewarded in the ISF Score.
Once the analysis is performed, we bucket the output in a few standardized categories that can be uniformly used for different AV stacks. While the output categories are standardized, the underlying metrics could be custom to each use case. If a given AV stack runs across both robotaxi and trucking deployments, it is highlighted in the output (that is why we score a “deployment”, not just a “stack”). Standardization allows us to generate scores that are interpretable, repeatable, and thus reliable from one risk to another, which we believe is a critical requirement for this kind of insurance analysis. With our proprietary scoring scheme, we generate a set of ISF Scores (or sub-scores, to be exact) for those categories, which get rolled up into the final ISF Score. The score is normalized across a certain scale, with a higher score meaning better risk assessment.
The purpose of the ISF Score is to serve as a practical tool to insure autonomous vehicles, which is quite challenging today. The insurance industry must innovate in ways that would live up to the requirements of its customer base, which in our case is revolutionizing transportation as we know it. ISF Score is currently piloting across different customer groups and is promising to become the tool that wouldn’t just live up to the expectations of our insureds but also outperform those expectations on the insurance front.
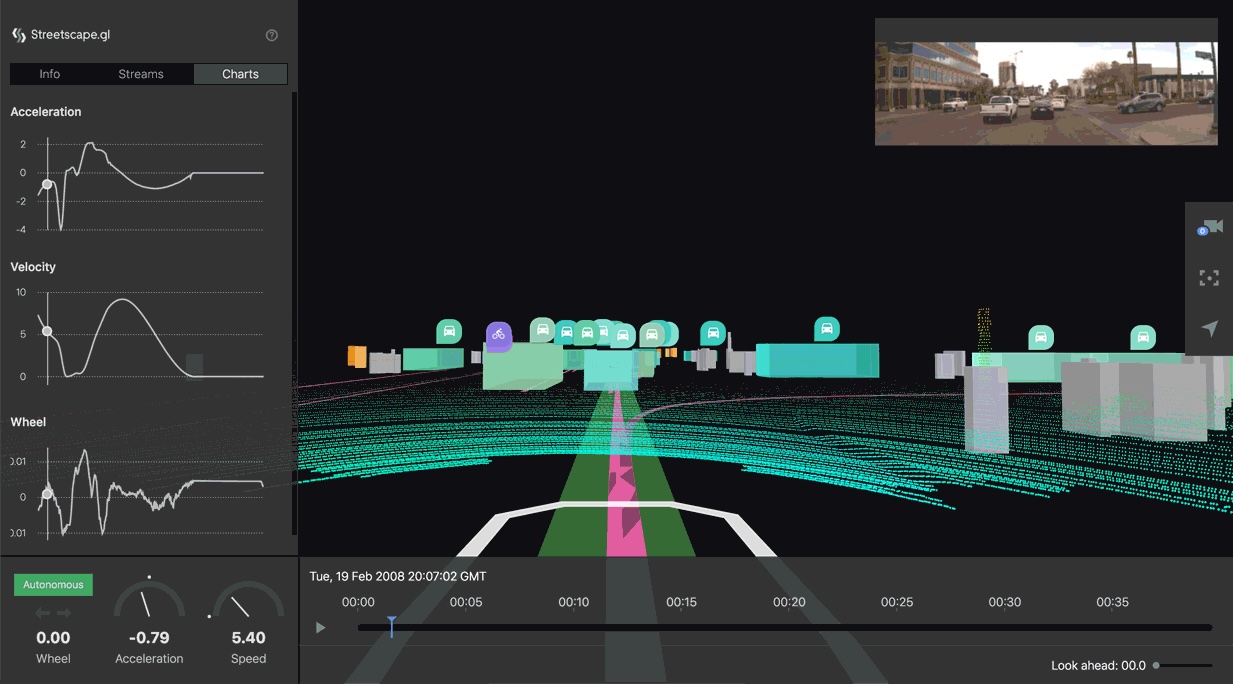
Rating
But just having a score is not enough. To fulfill our mission, we must be able to connect the score with how insurance coverage is rated. This must then align with the coverage language and policy structure itself. Traditional insurance options available in the market today are based on decades of human drivers’ experience and millions of miles of accident data. Insurance companies usually use their data as a competitive advantage when coming up with new insurance products. The problem for AV deployments is that this data is rooted in human risk or, in other words, with distributions of human errors on the road. Removing the human from behind the steering wheel (and soon having no steering wheel at all) makes it very challenging for insurers to go about determining rates and coverages that exemplify an understanding of the AV risk (and rightfully so). There is an expiration date on the mean frequency and severity of human-centric crash metrics and on the traditional auto/motor carrier coverages that assume that a driver can only be a human driver. The last thing that the AV industry wants is to ship a commercial autonomous product and not be able to scale it due to insurance issues.
We believe that the ISF Score is the answer to the gridlock described above. It provides the basis for rating that incorporates an AV-specific risk assessment into how insurance premium is calculated. In turn, that opens doors to new insurance product development, which we believe is much needed for the industry to stay competitive in the autonomous future. With ISF, we are able to provide an understanding of the risk that we believe is unmatched in the market. This, in turn, will allow for pricing that is developed for AVs and not weighted on human-operated experience. It will acknowledge safety engineering and operational excellence by criteria specific to this industry. In the end, it will produce insurance that will be rated for AVs substantially better than what exists today for a human driver.

In the following (and final) part of this ISF series, we will touch upon some of the industry-wide issues regarding insuring autonomous vehicles and share some of our near-term plans. In the meantime, please make sure to follow our progress on LinkedIn and sign up for company updates here. If you would like to learn more about Koop Technologies, feel free to check out our website or reach out to us directly at hello@koop.ai!
Previously in the ISF series: “Introducing ISF: Insurability Sufficiency Framework for Autonomous Vehicles” Part 1.